You signed in with another tab or window. Reload to refresh your session.You signed out in another tab or window. Reload to refresh your session.You switched accounts on another tab or window. Reload to refresh your session.Dismiss alert
논문에 따르면 모바일 환경을 위한 backbone 구조는 종종 FLOP 또는 파라미터 수와 같은 metric에 맞춰서 나오는데, 이러한 방식은 실제로 모바일 기기에 배포될 때 network latency가 잘 고려가 안된다고 하네요. 그래서 애플에서는 mobile friendly network 구조인 MobileOne backbone을 제안합니다. ImageNet에서 75.9%의 정확도로 iPhone 환경에서 1ms 미만의 추론 시간을 달성한다고 하네요. 본 논문에서 제안한 모델 중 best model은 MobileFormer 방법과 비교했을 때 유사한 성능을 보이면서 약 38배 정도 빠르다고 하네요. 그리고 비슷한 latency에서 EfficientNet 보다 2.3% 정도 더 정확하다고 합니다.
예를 들면 MobileOne-S1 구조에서는 4.8M개의 파라미터, 0.89ms의 latency가 발생하는 반면, MobileNet-V2 구조에서는 3.4M개의 파라미터를 가지지만(29.2% 적음) 0.98ms 만큼의 시간이 걸립니다. 즉, 파라미터 수가 적더라도 시간이 더 오래 걸리는 결과를 확인할 수 있습니다. 정확도도 MobileOne이 3.9% 정도 낫다고 합니다.
Main Contribution
모바일 장치에서 1ms 이내 실행되고, Image Classification에서 SOTA를 달성함, 데스크탑 CPU 및 GPU에서도 일반화 됨
모바일 환경에서 최근 등장한 신경망들이 왜 높은 latency를 가지는지 분석
re-parameterizable branch의 효과와 정규화의 동적 완화를 분석
Method
Key Bottlenecks
Activation Function
SE-ReLU 및 Dynamic Shift-Max, DynamicReLU와 같은 최근 활성화 함수들은 synchronization cost가 많이 든다고 합니다. DynamicReLU 및 Dynamic Shift Max는 MicroNet과 같은 매우 낮은 FLOP 모델에서 상당한 정확도 향상을 보여주었지만 시간이 상당히 들 수 있다고 합니다. 그래서 MobileOne 에서는 ReLU 만 사용합니다.
Architectural Blocks
runtime 성능에 영향을 미치는 두 가지 핵심 요소는 memory access cost와 병렬처리를 얼마나 하는가 입니다. multi branch 구조 같은 경우 graph의 다음 tensor를 계산하기 위해 각 branch의 activation을 저장해야 하기 때문에 memory access cost가 크게 증가한다고 합니다. 이러한 메모리 병목 현상은 신경망에서 분기를 최소화 하여 피할 수 있습니다. Squeeze-Excite block에서 사용되는 global pooling 과 같은 작업도 synchronization cost으로 인해 실행 시간에 영향을 미치게 됩니다.
그래서 본 논문에서는 skip connection 및 squeeze-excite block을 없앱니다. 이에 따른 표 3에 나타나 있습니다. 특히 이 SE block은 정확도를 향상시키기 위해 MobileOne의 가장 큰 버전에 들어간다고 합니다.
MobileOne Architecture
MobileOne Block
MobileOne Block은 depthwise 및 pointwise layer로 나눠지는 conv layer로 설계되었고, 정확도 향상을 위해 over parameterization branch를 도입합니다. basic block은 MobileNet-V1 3x3 depthwise conv 를 기반으로 합니다. 그 다음 그림 3과 같이 구조를 복제하는 branch와 함께 batchnorm을 사용하여 re-parameterizable skip connection을 도입합니다. inference 과정에서는 branch가 없습니다.
Model Scaling
본 논문에서는 5가지 width scale을 사용합니다.
Experiments
본 논문에서는 128 만 개의 학습 이미지와 1,000개 클래스의 50,000개 이미지로 구성된 validation 세트로 구성된 ImageNet 데이터 세트에서 MobileOne 모델을 평가합니다. 모든 모델은 8개의 NVIDIA GPU가 있는 시스템에서 PyTorch 라이브러리를 사용하여 스크래치 학습됩니다. 모든 모델은 Momentum optimizer와 함께 SGD를 사용하여 배치 크기 256으로 300 epoch 동안 학습됩니다.
또한 AutoAugment를 사용하여 MobileOne S2, S3, S4를 학습합니다. auto augmentation의 강도와 이미지 해상도는 논문[56]에 소개된 것처럼 학습 중에 점진적으로 증가합니다. MobileOne S0, S1 모델의 경우, standard augmentation(random resized cropping and horizontal flipping)을 사용합니다.
마이크로 버전 성능도 있습니다
CPU, GPU, TPU 환경에서의 성능은 아래와 같습니다.
이외에 부록에서는 MobileNet V3에서 H-swish 연산자가 특정 하드웨어 플랫폼에 최적화 되어있고 다른 플랫폼에서는 최적화 되어있지 않다고 언급하고 있습니다. Howardet al. [30] 논문에서는 H-swish가 플랫폼 별 최적화가 적용될 때 ReLU와 유사한 성능을 얻을 수 있다는 것을 입증했기에 H-swish layer를 ReLU layer로 대체하여 시간을 측정했다고 하네요. 부록에 볼만한 내용이 많은 것 같습니다.
Paper : https://arxiv.org/pdf/2206.04040.pdf
GitHub : https://github.com/apple/ml-mobileone
오늘 읽어볼 논문은 Apple에서 공개한 MobileOne이며, CVPR 2023에 소개될 내용이라고 하네요.
논문에 따르면 모바일 환경을 위한 backbone 구조는 종종 FLOP 또는 파라미터 수와 같은 metric에 맞춰서 나오는데, 이러한 방식은 실제로 모바일 기기에 배포될 때 network latency가 잘 고려가 안된다고 하네요. 그래서 애플에서는 mobile friendly network 구조인 MobileOne backbone을 제안합니다. ImageNet에서 75.9%의 정확도로 iPhone 환경에서 1ms 미만의 추론 시간을 달성한다고 하네요. 본 논문에서 제안한 모델 중 best model은 MobileFormer 방법과 비교했을 때 유사한 성능을 보이면서 약 38배 정도 빠르다고 하네요. 그리고 비슷한 latency에서 EfficientNet 보다 2.3% 정도 더 정확하다고 합니다.
예를 들면 MobileOne-S1 구조에서는 4.8M개의 파라미터, 0.89ms의 latency가 발생하는 반면, MobileNet-V2 구조에서는 3.4M개의 파라미터를 가지지만(29.2% 적음) 0.98ms 만큼의 시간이 걸립니다. 즉, 파라미터 수가 적더라도 시간이 더 오래 걸리는 결과를 확인할 수 있습니다. 정확도도 MobileOne이 3.9% 정도 낫다고 합니다.
Main Contribution
Method
Key Bottlenecks
Activation Function
SE-ReLU 및 Dynamic Shift-Max, DynamicReLU와 같은 최근 활성화 함수들은 synchronization cost가 많이 든다고 합니다. DynamicReLU 및 Dynamic Shift Max는 MicroNet과 같은 매우 낮은 FLOP 모델에서 상당한 정확도 향상을 보여주었지만 시간이 상당히 들 수 있다고 합니다. 그래서 MobileOne 에서는 ReLU 만 사용합니다.
Architectural Blocks
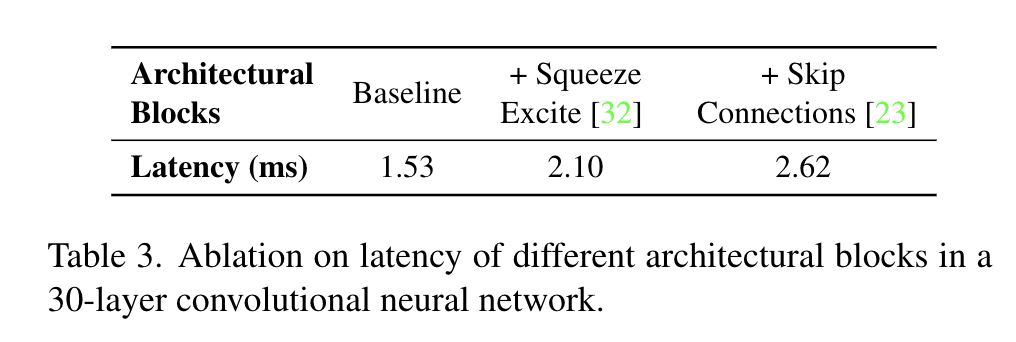
runtime 성능에 영향을 미치는 두 가지 핵심 요소는 memory access cost와 병렬처리를 얼마나 하는가 입니다. multi branch 구조 같은 경우 graph의 다음 tensor를 계산하기 위해 각 branch의 activation을 저장해야 하기 때문에 memory access cost가 크게 증가한다고 합니다. 이러한 메모리 병목 현상은 신경망에서 분기를 최소화 하여 피할 수 있습니다. Squeeze-Excite block에서 사용되는 global pooling 과 같은 작업도 synchronization cost으로 인해 실행 시간에 영향을 미치게 됩니다.
그래서 본 논문에서는 skip connection 및 squeeze-excite block을 없앱니다. 이에 따른 표 3에 나타나 있습니다. 특히 이 SE block은 정확도를 향상시키기 위해 MobileOne의 가장 큰 버전에 들어간다고 합니다.
MobileOne Architecture
MobileOne Block
MobileOne Block은 depthwise 및 pointwise layer로 나눠지는 conv layer로 설계되었고, 정확도 향상을 위해 over parameterization branch를 도입합니다. basic block은 MobileNet-V1 3x3 depthwise conv 를 기반으로 합니다. 그 다음 그림 3과 같이 구조를 복제하는 branch와 함께 batchnorm을 사용하여 re-parameterizable skip connection을 도입합니다. inference 과정에서는 branch가 없습니다.
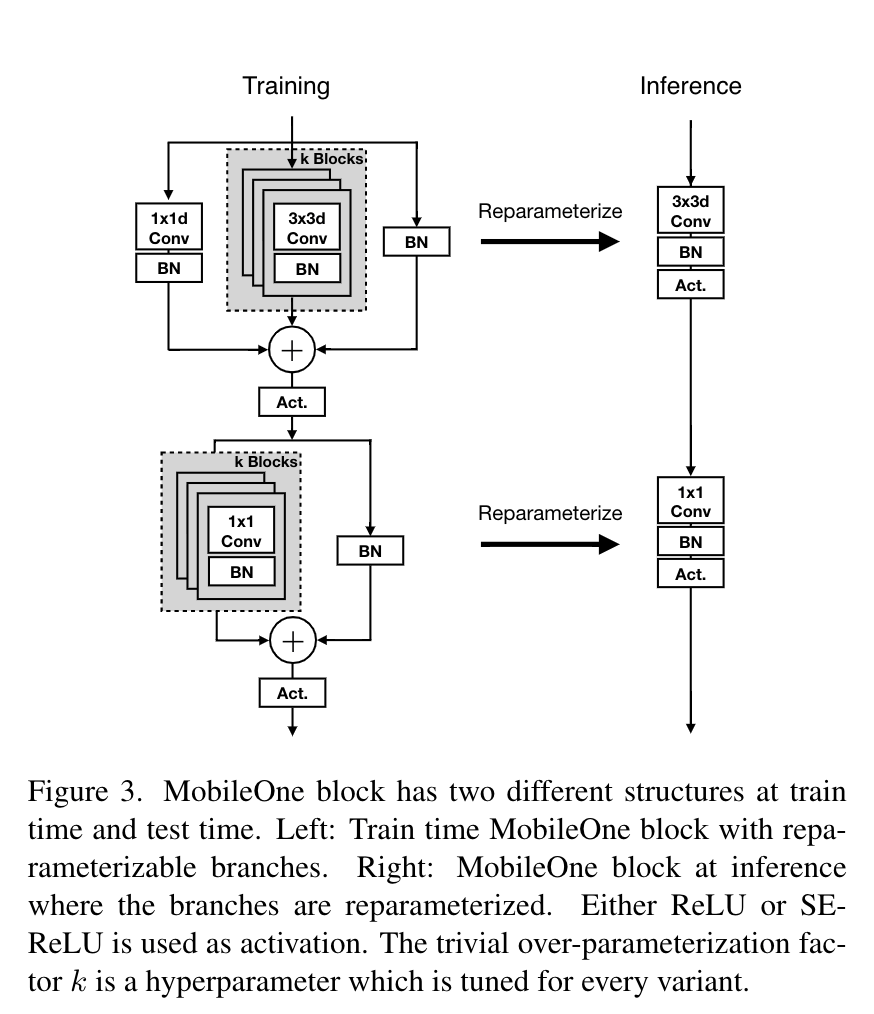
Model Scaling
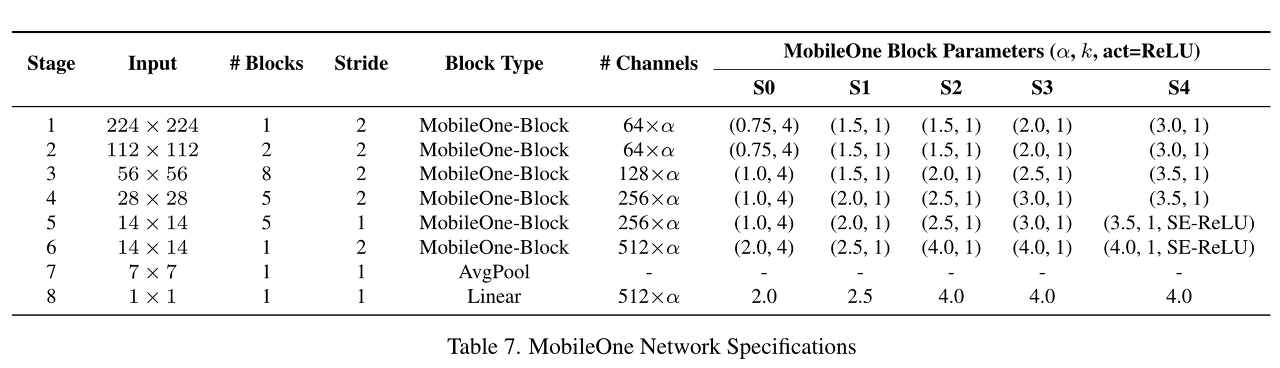
본 논문에서는 5가지 width scale을 사용합니다.
Experiments
본 논문에서는 128 만 개의 학습 이미지와 1,000개 클래스의 50,000개 이미지로 구성된 validation 세트로 구성된 ImageNet 데이터 세트에서 MobileOne 모델을 평가합니다. 모든 모델은 8개의 NVIDIA GPU가 있는 시스템에서 PyTorch 라이브러리를 사용하여 스크래치 학습됩니다. 모든 모델은 Momentum optimizer와 함께 SGD를 사용하여 배치 크기 256으로 300 epoch 동안 학습됩니다.
또한 AutoAugment를 사용하여 MobileOne S2, S3, S4를 학습합니다. auto augmentation의 강도와 이미지 해상도는 논문[56]에 소개된 것처럼 학습 중에 점진적으로 증가합니다. MobileOne S0, S1 모델의 경우, standard augmentation(random resized cropping and horizontal flipping)을 사용합니다.
마이크로 버전 성능도 있습니다
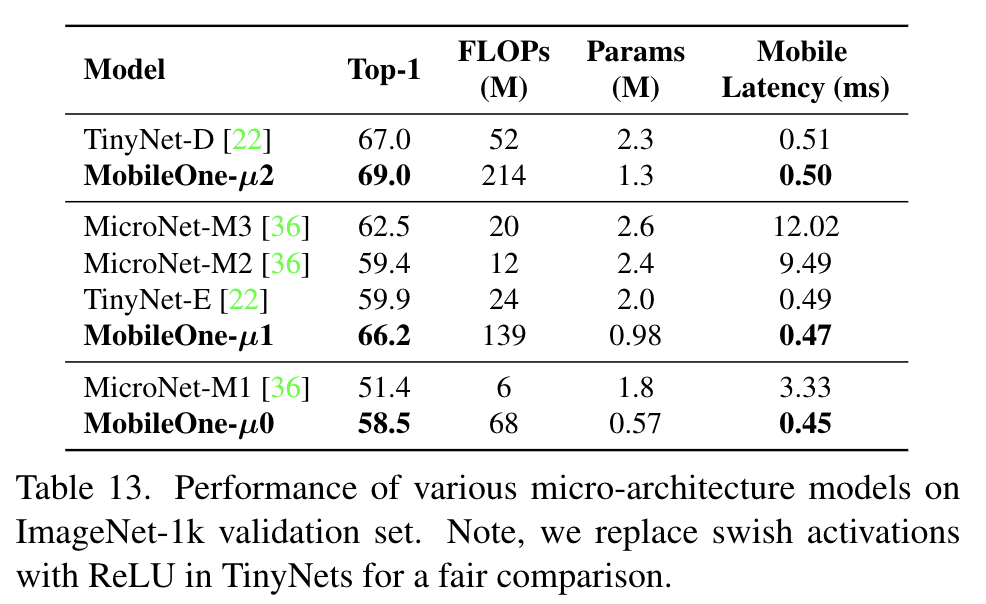
CPU, GPU, TPU 환경에서의 성능은 아래와 같습니다.
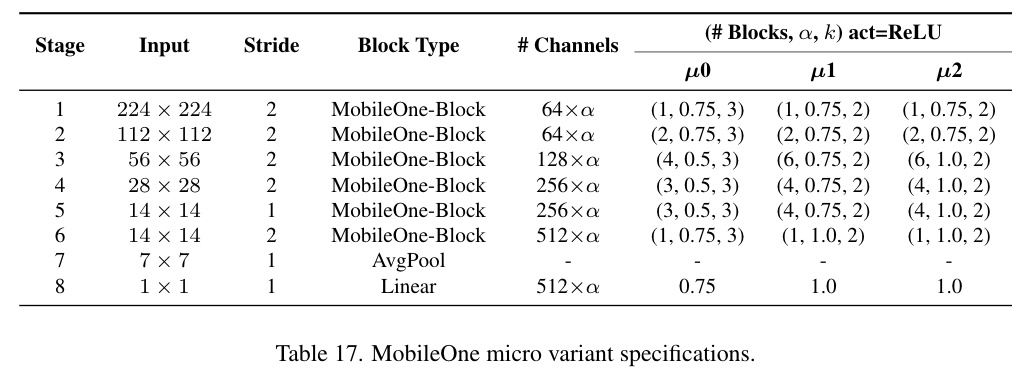
이외에 부록에서는 MobileNet V3에서 H-swish 연산자가 특정 하드웨어 플랫폼에 최적화 되어있고 다른 플랫폼에서는 최적화 되어있지 않다고 언급하고 있습니다. Howardet al. [30] 논문에서는 H-swish가 플랫폼 별 최적화가 적용될 때 ReLU와 유사한 성능을 얻을 수 있다는 것을 입증했기에 H-swish layer를 ReLU layer로 대체하여 시간을 측정했다고 하네요. 부록에 볼만한 내용이 많은 것 같습니다.
Full version : https://eehoeskrap.tistory.com/702
The text was updated successfully, but these errors were encountered: