You signed in with another tab or window. Reload to refresh your session.You signed out in another tab or window. Reload to refresh your session.You switched accounts on another tab or window. Reload to refresh your session.Dismiss alert
Is there a current way to change the Z-order of the plot? I would like the plot below to have the green lines plot on top of the (almost) transparent ones.
Currently, I am subclassing QuantitativeScale as in the docs to only color based on the source. Perhaps it's possible to turn off the links somewhere as well?
The text was updated successfully, but these errors were encountered:
Is there a current way to change the Z-order of the plot? I would like the plot below to have the green lines plot on top of the (almost) transparent ones.
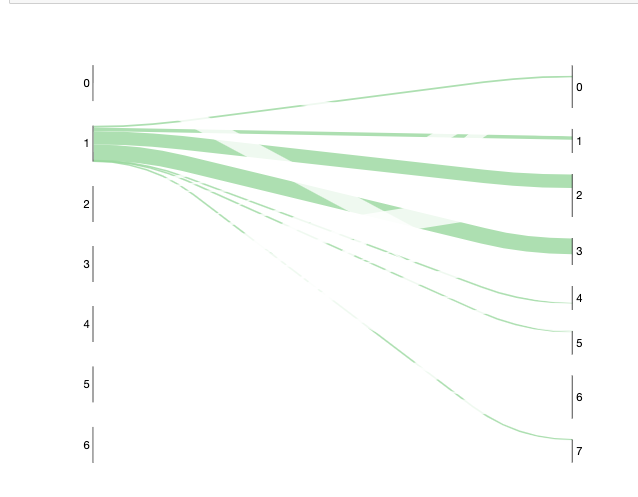
Code:
Currently, I am subclassing QuantitativeScale as in the docs to only color based on the source. Perhaps it's possible to turn off the links somewhere as well?
The text was updated successfully, but these errors were encountered: