-
Notifications
You must be signed in to change notification settings - Fork 0
/
Copy pathplan
521 lines (356 loc) · 13.3 KB
/
plan
1
2
3
4
5
6
7
8
9
10
11
12
13
14
15
16
17
18
19
20
21
22
23
24
25
26
27
28
29
30
31
32
33
34
35
36
37
38
39
40
41
42
43
44
45
46
47
48
49
50
51
52
53
54
55
56
57
58
59
60
61
62
63
64
65
66
67
68
69
70
71
72
73
74
75
76
77
78
79
80
81
82
83
84
85
86
87
88
89
90
91
92
93
94
95
96
97
98
99
100
101
102
103
104
105
106
107
108
109
110
111
112
113
114
115
116
117
118
119
120
121
122
123
124
125
126
127
128
129
130
131
132
133
134
135
136
137
138
139
140
141
142
143
144
145
146
147
148
149
150
151
152
153
154
155
156
157
158
159
160
161
162
163
164
165
166
167
168
169
170
171
172
173
174
175
176
177
178
179
180
181
182
183
184
185
186
187
188
189
190
191
192
193
194
195
196
197
198
199
200
201
202
203
204
205
206
207
208
209
210
211
212
213
214
215
216
217
218
219
220
221
222
223
224
225
226
227
228
229
230
231
232
233
234
235
236
237
238
239
240
241
242
243
244
245
246
247
248
249
250
251
252
253
254
255
256
257
258
259
260
261
262
263
264
265
266
267
268
269
270
271
272
273
274
275
276
277
278
279
280
281
282
283
284
285
286
287
288
289
290
291
292
293
294
295
296
297
298
299
300
301
302
303
304
305
306
307
308
309
310
311
312
313
314
315
316
317
318
319
320
321
322
323
324
325
326
327
328
329
330
331
332
333
334
335
336
337
338
339
340
341
342
343
344
345
346
347
348
349
350
351
352
353
354
355
356
357
358
359
360
361
362
363
364
365
366
367
368
369
370
371
372
373
374
375
376
377
378
379
380
381
382
383
384
385
386
387
388
389
390
391
392
393
394
395
396
397
398
399
400
401
402
403
404
405
406
407
408
409
410
411
412
413
414
415
416
417
418
419
420
421
422
423
424
425
426
427
428
429
430
431
432
433
434
435
436
437
438
439
440
441
442
443
444
445
446
447
448
449
450
451
452
453
454
455
456
457
458
459
460
461
462
463
464
465
466
467
468
469
470
471
472
473
474
475
476
477
478
479
480
481
482
483
484
485
486
487
488
489
490
491
492
493
494
495
496
497
498
499
500
501
502
503
504
505
506
507
508
509
510
511
512
513
514
515
516
517
518
519
520
521
# Goals
- Learn about the need of reproducibility
- Know how to improve the organisation of your (R) projects
- Make your code cleaner, documented and shorter
- Learn how to track changes in your code
# Program
- **Introduction (20 minutes):**
- Welcome
- Importance of reproducibility in epidemiology research
- The principles of a reproducible workflow
- Creating and organizing a project structure for reproducibility
- Avoiding copy pasting with functions
- Git basic to keep track your code
- Real-world examples and case studies
- **Hands-on Activity 1: a simple reproducible project (40 minutes)**
- How to organize project's files
- Optimize the analysis with functions
- Add comments and style the scripts
- **Break (30 minutes)**
- **Hands-on Activity 2: Reporting and Git (50 minutes)**
- Create a nice report for your results
- Make nice tables using `gt`
- Use to git to save your progress
- **Closing Remarks and Q&A (15 minutes)**
- Summary of key takeaways
- Resources for further learning
- Open floor for questions and discussion
# Theory
Reproducibility
: Obtain consistent results using the **same data and code** as the original study
Replicability
: Obtain consistent results **across studies** aimed at answering the same scientific question using **new data** or other new computational methods.
::: footer
@nationalacademiesofsciences2019
:::
:::notes
Unreplicability can be useful to science and help build knowledge.
- Domain of study
- Complex / unknown systems
- Researchers choices:
- **Study design**
- **Mistakes**
- **Misconduct**
- **Poor reporting and publication bias**
Source of not useful non-replication:
- publication bias
- misaligned incentives
- inappropriate statistical inference
- poor study design
- errors
- incomplete reporting of a study
:::
Related to data collection, computation, and analysis:
- data acquisition,
- data management,
- analysis,
- report of result...
::: callout-important
Almost all the steps of an epidemiology study can be involved!
:::
{fig-alt="reproducibility spectrum" fig-align="center"}
::: footer
@peng2011
:::
Workshop:
- focus on files organisation
- coding practices
- documentation
- tracking of changes
# Project
project: many definitions
one analysis for a paper, a entire cohort with differents papers
usullay refer to a folder on your computer
Suggest :
- make project related to a dataset
- usally one papers wiht one analysis
Starting the workshop project:
- Open R studio
- Create proejct in empty directory
- Use a relevant name: isee-young-ws
In the setup configure project option: saving working data (best to disable), see other options:
Advantages:
working directory is set by R studio as project folder.
easy to switch between project from the drop down menu
img/rstudio-project.png
https://support.posit.co/hc/en-us/articles/200526207-Using-RStudio-Projects
# Files organisation
Not a single organisation fits all needs. But try to be consistant throught different project / research groups
Since working with custome directory, no need for sp
## Directory
- `data/` Ready-to-analyze dataset, intermediate datasets.
- `data-raw/` Data from the outside world untouched. Can contains scripts to import data from internet and perpare it.
- `R/` R files containing functions, easy to used them in many documents. `python/` for python
- `scripts/` `code/` for script of things than need to be runned once
- `qmd/`, `md/`, `Rmd/` quarto and markdown documents
- `output/`: folder with outputs, can be images, graph or other stuff
- `figs/`: folder with figure produced by your scripts
- `results/` results from the project, ex: csv tables
- `docs/`: documentation or rendered documents
- `man/`: documentation for R pacakges
- `extra/`: Extra, non-code, files
https://rfortherestofus.com/2021/08/rstudio-project-structure
https://www.stat.ubc.ca/~jenny/STAT545A/block19_codeFormattingOrganization.html
https://joshua.wilsonblack.nz/post/organising-r-projects/
## Usefull files to add
- `README`: Must read file. A least one in the project directory, but can be added to any folder
- `DESCRIPTION`
- `LICENSE`: License file
- `.gitignore`: List files that git should ignore
``` text
data-raw/
├── README.md
├── mmash
│ ├── user_1
│ ├── user_10
│ ├── user_11
│ ├── ...
│ ├── user_7
│ ├── user_8
│ └── user_9
├── mmash-data.zip
└── mmash.R
```
## Naming files
human readable
machine readable
easily sortable
- Dates : YYYY-MM-DD (iso format)
- Easy to erder, use double digits
- separate names element to make them easy to subset with `r str_sub()`: `model_1`
- regular expression and globbing friendly: avoid spaces, punctuation, accented , characters, case sensitivity
- easy to compute on: deliberate use of delimiters
ex for data:
2024-04-01_air-pollution_PM25.csv
2024-04-01_air-pollution_NO2.csv
2021-04-01_air-pollution_PM25.csv
2021-04-01_air-pollution_NO2.csv
try this
```{r}
file.create("2024-04-01_air-pollution_PM25.csv")
file.create("2024-04-01_air-pollution_NO2.csv")
file.create("2021-04-01_air-pollution_PM25.csv")
file.create("2021-04-01_air-pollution_NO2.csv")
list.files(pattern = "2024*PM")
```
```
# Bad
alternative model.R
code for exploratory analysis.r
finalreport.qmd
FinalReport.qmd
Filenames Use Spaces and Punctuation.xlsx
fig 1.png
Figure_02.png
figure 1.png
fig 2.png
JW7d^(2sl@deletethisandyourcareerisoverWx2*.txt
model_first_try.R
myabstract.docx
run-first.r
temp.txt
```
```
# Good
other/2014-06-08_abstract-for-sla.docx
other/1986-01-28_raw-data-from-challenger-o-rings.txt
other/filenames-are-getting-better.xlsx
01-load-data.R
02-exploratory-analysis.R
03-model-approach-1.R
04-model-approach-2.R
fig-01.png
fig-02.png
fig01_scatterplot-talk-length-vs-interest.png
fig02_histogram-talk-attendance.png
report-2022-03-20.qmd
report-2022-04-02.qmd
report-draft-notes.txt
```
https://r4ds.hadley.nz/workflow-scripts#saving-and-naming
https://rstats.wtf/source-and-blank-slates
# Iprove R code
## style guide
why adopting a style:
consistency
making code easier to write because you need to make fewer decisions
Tydyverse style guide:
https://style.tidyverse.org/
### Syntax:
- Variable and function names:
- only lowercase letters,
- numbers,
- `_` to separate words within a name
`day_one day_1`
-
- space after a coma `mean(x, na.rm = TRUE)`
2.4.1 Code blocks
Curly braces, {}, define the most important hierarchy of R code. To make this hierarchy easy to see:
{ should be the last character on the line. Related code (e.g., an if clause, a function declaration, a trailing comma, …) must be on the same line as the opening brace.
The contents should be indented by two spaces.
} should be the first charac
Quickly fix the syntax of your code using `styler`:

### Naming r object names
- avoid changing commons R function or objects
```{r}
#| eval: false
T <- "true"
c <- "true"
sum <- function(x) mean(x)
```
- use verbs for functions : `do_somethings()`
- new line after a pipe (`%>%` or `|>`)
Prefer using element name to retrives object index instead of number indexing
````{r}
#| eval: false
# Bad
iris[, c(2,4)]
mod <- lm(Sepal.Width ~ Petal.Width, data = iris)
mod[[1]][2]
```
````{r}
#| eval: false
# Good
mod$coefficients[names(mod$csoefficient) == "Petal.Width"]
iris[, c("Sepal.Width", "Petal.Width")]
iris[, grep("Width", colnames(iris))]
```
comment as you code, details as much as you can. Your furture you will thanks you.
```{r}
#| eval: false
# Better
# Inspect iris data
iris[, grep("Width", colnames(iris))]
# Linear regression between sepal and petal width
mod <- lm(Sepal.Width ~ Petal.Width, data = iris)
# Beta from the regression for petal width
# Low correlation
mod$coefficients[names(mod$coefficient) == "Petal.Width"]
```
# Git
## But Word works fine
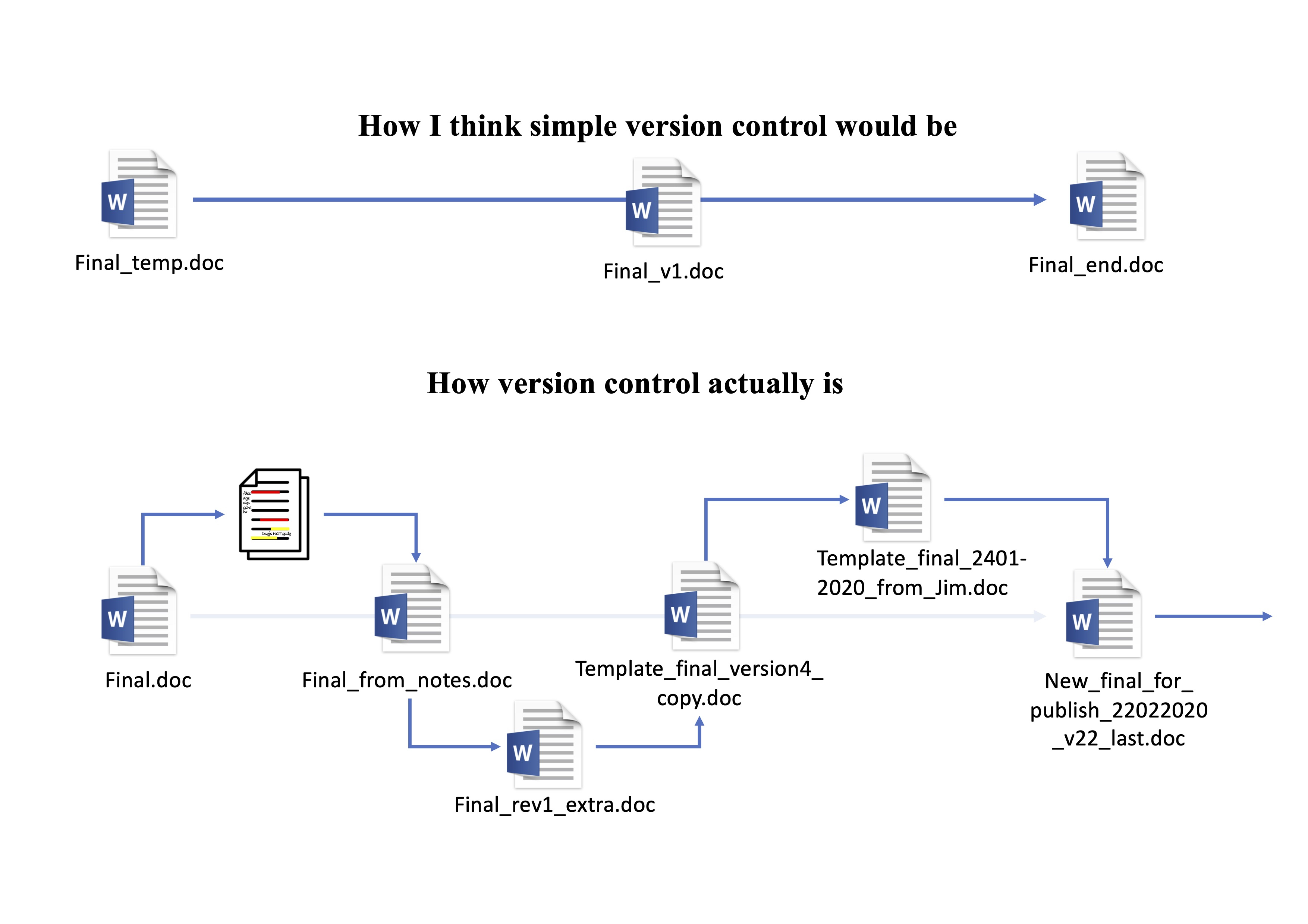
Manual version control system, Luke W. Johnston
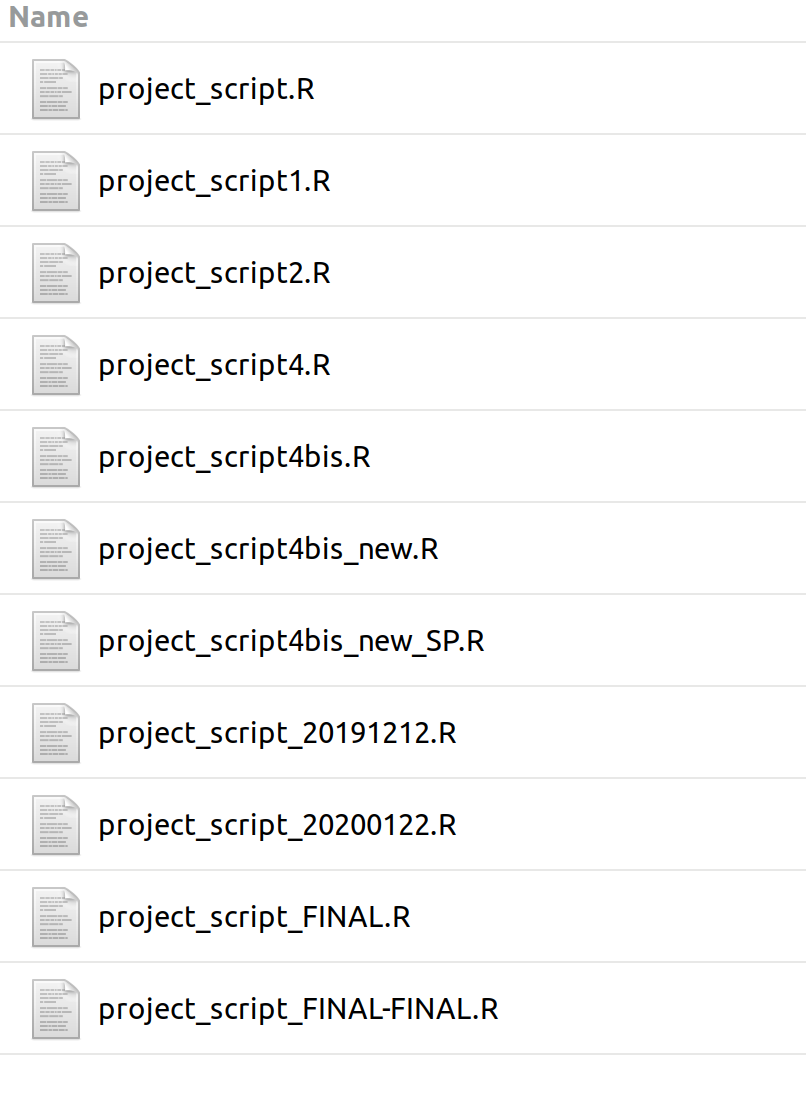
## Version control
Will keep track of every change to a file.
Can instantly restore any previous saved file.
Make you able to split the work in diffrent branches if you don't want ot crash
Handle merging files by comparing their modifications and resolve differences if any (similar to compare and merge from word)
::: {.callout-tip}
With git you only need one version of your project files!
:::
## Which files
Tracks mostly text files:
- R Script
- csv data
- markup documents like markdown, quarto of latex files
Best to setup git for a whole project, eg by folder.
## Terms to know
```{mermaid fig-git-states}
%%| label: fig-git-states
%%| fig-cap: The three states that files and folders can be in, when using Git.
%%| echo: false
%%| eval: true
%%{init:{'themeCSS': ".actor {stroke: DarkBlue;fill: White;stroke-width:1.5px;}", 'sequence':{'mirrorActors': false}}}%%
sequenceDiagram
participant W as Working folder
participant S as Staged
participant H as History
W->>S: Add
S->>H: Commit
```
- `repository`, `repo`: a folder tracked by git
- `working folder`: files here are no tracked by git, or contains new modification not save yet
- `add`: add files of modifications to be tracked by git
- `stage`: files here are tracked byt git and can be put into the history with a `commit`.
- `commit`: create a snapshot of changes and save it in git `history`. Commits can have a short description of the changes
- `history`: stored all the changes that have been `commited`. Everything that has been commited into the Git history will never be completely gone
- `local`: refer to the repository that you store on your computer
- `remote`: refer to the repository that are stored online like git hub
- `branch`: branches are parallel versions of your project. They allow you to experiment things without affecting the main project until you’re ready to merge them back.
- `merge`: merging is the process of integrating changes from one branch into another. It combines the histories of both branches, creating a single, unified history
## Files added by git
```text
Project
├── .git/ <-- Git repository stored here (eg data about changes)
├── R/
├── data/
├── data-raw/
├── doc/
├── .gitignore <-- Tells Git which files NOT to save
├── LearningR.Rproj
├── DESCRIPTION
└── README.md
```
## Configure git for the workshop project
```{bash}
# GIT bash
git config --global user.name "First Last"
git config --global user.email "first.last@example.com
```
Can also be created using Rstudio project by selecting `Create a git repository` option in the `New project` menu.
RStudio also has a interfac for git but it can be limited sometimes.
# Writing functions
Why?
Don’t repeat yourself.
Golden rule of programming
- Create function for things that you will do often in a similar maner:
- create models
- extract results from a model
- create tables
Output of the function depend only on their imputs. Return same result with same inputs
Can replace loops, and make your code much more clearer.
Functions can be sahred between project, can be gathered in a package
## Tools
Open source
: Software or code that is made freely available for possible modification and **redistribution**.
- Software
- Word vs **Libre Office**,
- Stata or SAS vs **R**
- Windows vs **Linux**
- iOS vs **Android**
Why open source matter:
- Code can be shared or modified easily with version control systems (ex: git)
- Easy to install the same software and run the same code on another computer (ex: virtual machine)
{fig-align="center" width="321"}
Beside analysis code and data, manuscripts and papers can also be more reproducible:
- Collaborative and text based editors (Google Doc, Overleaf...)
- Insert results directly from the code (RMarkdown, Quarto, gt tables...)
- Publication on preprint (ArXiv, bioRxiv...)
## Check list
{fig-alt="reproducibility checklist" fig-align="center"}
::: footer
@alston2021
:::
- Welcome
- Importance of reproducibility in epidemiology research
- The principles of a reproducible workflow
- Creating and organizing a project structure for reproducibility
- Avoiding copy pasting with functions
- Git basic to keep track your code
- Real-world examples
## To go further {.smaller .scrollable}
- Papers:
- "Reproducibility Project: Cancer Biology", @scienceReproducibilityProjectCancer
- "Is science really facing a reproducibility crisis, and do we need it to?", @fanelli2018
- Tools:
- R:
- https://cran.r-project.org/
- https://posit.co/products/open-source/
- Git: https://ohmygit.org/, https://happygitwithr.com/
- Related course:
- [Reproducible Research in R](https://r-cubed-intro.rostools.org/sessions/introduction.html)
- [Harvard Free Courses - Reproducible research](https://pll.harvard.edu/course/principles-statistical-and-computational-tools-reproducible-data-science)
## References {.smaller}